OpenAI releases Point-E, artificial intelligence for 3D modeling. OpenAI, the AI startup founded by Elon Musk behind the popular DALL-E text image generator, has announced the release of its new AI for Point-E image creation, which can produce 3D dot clouds directly from prompts of text.
Samsung prioritizes OLEDs for 2024 iPad Pro
While existing systems like Google’s DreamFusion typically require multiple hours – and GPUs – to generate the images, Point-E only needs a GPU and a minute or two. 3D modeling is used in a variety of industries and applications. From the CGI effects of modern cinematic blockbusters, to video games, not to mention virtual reality and AR, NASA lunar crater mapping missions, Meta’s vision for the Metaverse.
All of these applications depend on 3D modeling capabilities. However, creating photorealistic 3D images is still a resource and time-consuming process, despite the work of NVIDIA to automate object generation and Epic Games’ RealityCapture mobile app, which allows anyone with an iOS smartphone to scan real-world objects and turn them into 3D images.
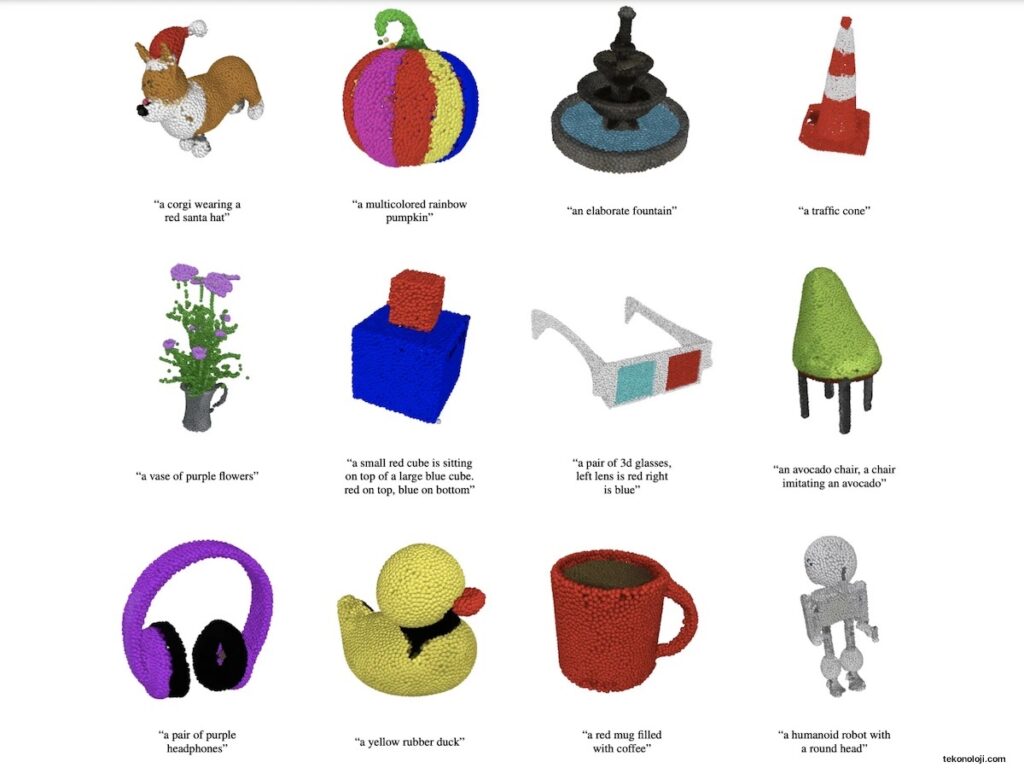
Text-to-Image systems like OpenAI and Craiyon’s DALL-E 2, DeepAI, Prisma Lab’s Lensa or HuggingFace’s Stable Diffusion, have rapidly gained popularity, notoriety (but also infamy) in recent years. These are resources that allow the creation of automated images starting from simple descriptions. How Point-E works is easy to say:
To produce a 3D object from a text prompt, we first sample an image using the text-to-image model, then sample a conditioned 3D object from the sampled image. Both of these steps can be performed in a number of seconds and do not require costly optimization procedures
Everything translates into an extremely simple use. It will be sufficient to write any description to allow the Point-E artificial intelligence to generate a synthetic 3D vision of what is requested. The system will take what you write and use a series of diffusion models to create the 3D and RGB dot cloud, producing a 1,024 point cloud model, then a more refined 4,096 point cloud.
These diffusion models have been trained on “millions” of 3D models, all converted into a standardized format. In all honesty, the team is aware that this system will lead to worse results than more advanced techniques, but it has the advantage of returning samples to the user in a small fraction of the time.